A groundbreaking new statistical method developed by MIT researchers has the potential to revolutionize forecasting precision in various fields, including climate research and public health.
A team of researchers from MIT has developed a new approach for evaluating predictions with a spatial dimension, such as forecasting weather or mapping air pollution. ‘The method could help scientists make more accurate forecasts in various fields.’
Spatial prediction problems involve predicting the value of a variable at a new location based on known values at other locations. Traditional validation methods often fail to accurately assess these predictions, leading to potentially misleading results. MIT researchers have shown that two classical methods can be substantively wrong when applied to spatial problems.
The Problem with Traditional Validation Methods
Traditional validation methods involve verifying data accuracy through manual checks and comparisons.
These methods include data entry verification, where human reviewers check entered data against original sources.
Another approach is data reconciliation, which involves identifying discrepancies between different datasets.
Traditional validation also includes data profiling, which analyzes data distributions to detect anomalies.
Additionally, data sampling allows for the evaluation of a subset of data to infer broader trends.
These methods are often used in conjunction with one another to ensure data accuracy and integrity.
Traditional validation methods assume that the data used for evaluation and the data being predicted are independent and identically distributed. However, in many spatial applications, this is not the case. For instance, air pollution sensors may be sited based on the location of other sensors, making them non-independent.
“This can lead to inaccurate assessments of predictive methods and potentially misleading conclusions.’
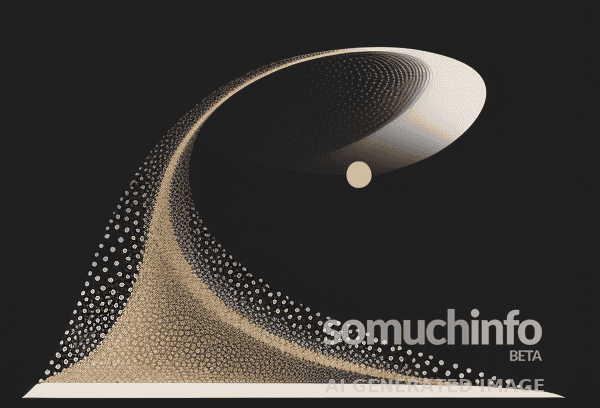
The researchers found that these assumptions break down when evaluating predictions with a spatial dimension. This can lead to inaccurate assessments of predictive methods and potentially misleading conclusions.
A New Approach
To address this issue, the MIT team developed a new method that assumes validation data and test data vary smoothly in space. ‘This regularity assumption is appropriate for many spatial processes and allows for more accurate evaluations of predictive methods.’
The researchers used their technique to evaluate two classical methods and found that it provided more accurate validations than these traditional techniques. They also applied the method to realistic problems, such as predicting wind speed at the Chicago O-Hare Airport and forecasting air temperature at five U.S. metro locations.
Potential Applications
The new validation technique could be applied to a range of problems, including climate research, public health, and ecological management. ‘It has the potential to improve uncertainty quantification in spatial settings and may also be useful for other areas where the regularity assumption is applicable, such as time-series data.’
The researchers plan to apply their techniques to improve predictive methods and find other areas where the regularity assumption could improve performance. The research was funded in part by the National Science Foundation and the Office of Naval Research.