Nvidia is betting big on synthetic data, acquiring startup Gretel to bolster its AI training data and tackle the challenges of scalable AI training.
Nvidia has acquired Gretel, a synthetic data startup, to bolster the AI training data used by the chip maker’s customers and developers.
The acquisition price exceeds Gretel’s most recent valuation of $320 million, according to two people with direct knowledge of the deal. The technology will be deployed as part of Nvidia’s growing suite of cloud-based, generative AI services for developers.
In theory, synthetic data could create a near-infinite supply of AI training data and help solve the data scarcity problem that has been looming over the AI industry since ‘ChatGPT went mainstream in 2022.’
Synthetic data refers to artificially generated data that mimics real-world data in terms of structure and statistical properties.
It is used to augment or replace existing datasets, particularly when actual data is scarce, biased, or sensitive.
Synthetic data can be created using various algorithms and techniques, including generative models and statistical modeling.
This type of data has several applications, such as improving machine learning model performance, protecting user privacy, and enhancing data analytics capabilities.
Nvidia has already been offering synthetic data tools for developers for years. In 2022 it launched Omniverse Replicator, which gives developers the ability to generate custom, physically accurate, synthetic 3D data to train neural networks.
NVIDIA is a global technology company that specializes in designing graphics processing units (GPUs) and high-performance computing hardware.
Founded in 1993, NVIDIA has become a leader in the fields of artificial intelligence (AI), deep learning, and computer vision.
Their GPUs are used in gaming, professional visualization, and data centers, while their AI technologies have applications in autonomous vehicles, healthcare, and finance.
Last June, Nvidia began rolling out a family of open AI models that generate synthetic training data for developers to use in building or fine-tuning LLMs.
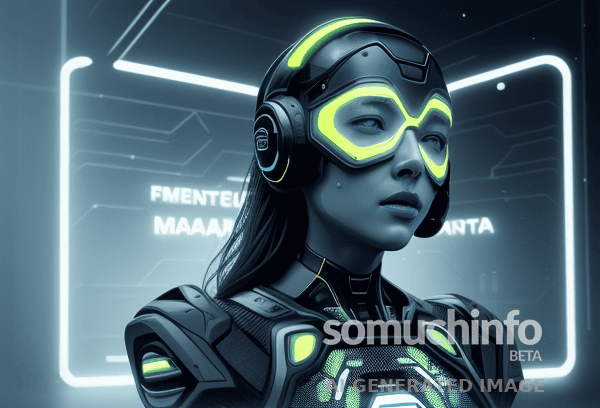
Synthetic data can be used in at least a couple different ways. It can take the form of tabular data, like demographic or medical data, which can solve a data scarcity issue or create a more diverse dataset.
However, experts say using synthetic data in generative AI comes with its own risks. In theory, if you feed the machine nothing but its own machine-generated output, it theoretically begins to eat itself, spewing out detritus as a result.
Ana-Maria Cretu, a postdoctoral researcher at the École Polytechnique Fédérale de Lausanne in Switzerland, who studies synthetic data privacy. She notes that most researchers and computer scientists are training on a mix of synthetic and real-world data. ‘You might possibly be able to get around model collapse by having fresh data with every new round of training,’ she says.
Concerns about model collapse haven’t stopped the AI industry from hopping aboard the synthetic data train, even if they’re doing so with caution. Big Tech has also been turning to synthetic data. Meta has talked about how it trained Llama 3, its state-of-the-art large language model, using synthetic data, some of which was generated from Meta’s previous model, Llama 2.
Alexandr Wang, the chief executive of Scale AI—which leans heavily on a human workforce for labeling data used to train models—shared the findings from the Nature article on X, writing, “While many researchers today view synthetic data as an AI philosopher’s stone, there is no free lunch.”
Alexandr Wang is a renowned American computer scientist and researcher specializing in artificial intelligence.
He is the founder of Scale AI, a leading platform for data labeling and annotation.
Wang received his Bachelor's degree from Stanford University and Ph.D. from MIT.
His research focuses on deep learning and natural language processing.
He has published numerous papers on AI-related topics and has been recognized with several awards for his contributions to the field.
He said later in the thread that this is why he believes firmly in a hybrid data approach.
The scientific theory around model collapse is sound. But it remains to be seen whether synthetic data can provide an easy solution to the challenges of scalable AI training. As the industry continues to evolve, one thing is clear: synthetic data will play a significant role in shaping the future of AI development.
- wired.com | Nvidia Bets Big on Synthetic Data