Revolutionizing Visual Generation: A New Approach Outperforms Industry Standards with AI-Powered Hybrid Autoregressive Transformer
The ability to generate high-quality images quickly is crucial for producing realistic simulated environments that can be used to train self-driving cars to avoid unpredictable hazards, making them safer on real streets. However, the generative artificial intelligence techniques increasingly being used to produce such images have drawbacks.
Self-driving cars, also known as autonomous vehicles, are equipped with advanced sensors and software that enable them to navigate roads without human input.
These vehicles use a combination of GPS, lidar, radar, and cameras to detect and respond to their environment.
According to the International Transport Forum, 40% of all miles driven in the US could be covered by self-driving cars, reducing traffic congestion and improving road safety.
Many major tech companies, such as Waymo and Tesla, are already testing and deploying autonomous vehicles on public roads.
Researchers from MIT and NVIDIA developed a new approach that brings together the best of both popular methods: an autoregressive model and a diffusion model. Their hybrid image-generation tool uses an autoregressive model to quickly capture the big picture and then a small diffusion model to refine the details of the image.
The new image generator, called HART (short for Hybrid Autoregressive Transformer), can generate images that match or exceed the quality of state-of-the-art diffusion models, but do so about nine times faster. The generation process consumes fewer computational resources than typical diffusion models, enabling HART to run locally on a commercial laptop or smartphone.
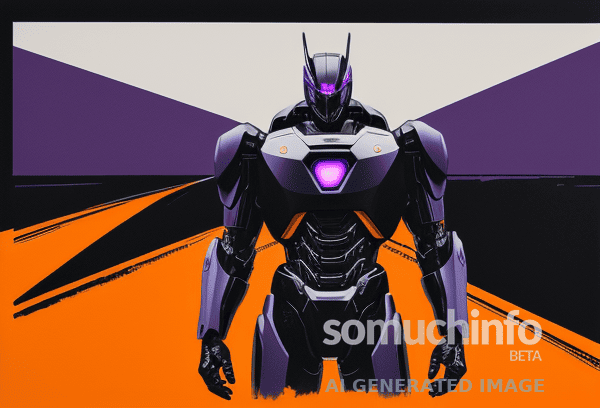
HART combines the strengths of both autoregressive and diffusion models. An autoregressive model is used to predict compressed, discrete image tokens, while a small diffusion model predicts residual tokens that compensate for the model’s information loss. This approach allows HART to achieve a huge boost in terms of reconstruction quality, with the diffusion model only needing to predict the remaining details after the autoregressive model has done its job.
During the development of HART, researchers encountered challenges in effectively integrating the diffusion model to enhance the autoregressive model. However, their final design of applying the diffusion model to predict only residual tokens as the final step significantly improved generation quality. The method uses a combination of an autoregressive transformer model with 700 million parameters and a lightweight diffusion model with 37 million parameters.
HART has wide range of applications, such as helping researchers train robots to complete complex real-world tasks and aiding designers in producing striking scenes for video games. The researchers also want to build vision-language models on top of the HART architecture and apply it for video generation and audio prediction tasks.
The Hybrid Autoregressive Transformer (HART) is a breakthrough in visual generation that leverages the strengths of both autoregressive and diffusion models. By combining these two approaches, HART can generate high-quality images quickly, making it an essential tool for various applications in computer vision, robotics, and design.