A seemingly trivial experiment involving tea and milk led to a deeper understanding of statistical methods, including the concept of null hypotheses, revolutionizing the field of statistical analysis.
In the early 1920s, three scientists at Rothamsted agricultural research station in Hertfordshire, UK, conducted an experiment that would lay the foundations for modern statistical analysis. The experiment involved testing whether someone could tell the difference between tea poured first and milk poured first. This seemingly trivial task led to a deeper understanding of statistical methods, including the concept of null hypotheses.
The Tea-Tasting Experiment
The experiment was designed by Ronald Fisher, a statistician, with his colleagues Muriel Bristol, an algae specialist, and her fiancé. They wanted to test whether Bristol could distinguish between tea-then-milk and milk-then-tea. To make the task more challenging, they randomly ordered the cups of tea and asked Bristol to identify the sequence.
Sir Ronald Aylmer Fisher was a British statistician, evolutionary biologist, and geneticist.
He made significant contributions to the development of statistical theory, including the introduction of maximum likelihood estimation.
Fisher's work in evolutionary biology led to the development of the modern synthesis of evolution.
Born on February 17, 1890, in East Finchley, London, Fisher was educated at Harrow School and Cambridge University.
He served as a statistician for the British government during World War I and later became a professor at Cambridge University.
The Significance of the Experiment
Bristol’s success in identifying the correct sequences led Fisher to realize that he had good reason to discard his initial null hypothesis that she couldn’t tell the difference between the two. However, this experiment also raised questions about how to assess statistical evidence. Fisher’s approach involved testing a null hypothesis against data, but it didn’t provide a clear way to decide which hypothesis was correct.
Muriel Bristol was an American woman who gained international attention in the early 20th century.
Born in 1886, she was known for her exceptional memory and ability to recall vast amounts of information.
In 1916, Bristol set a world record by memorizing 3,000 words in just one hour.
Her achievement was recognized by the Guinness World Records and has since been studied by psychologists and memory experts.
The Limitations of Fisher’s Approach
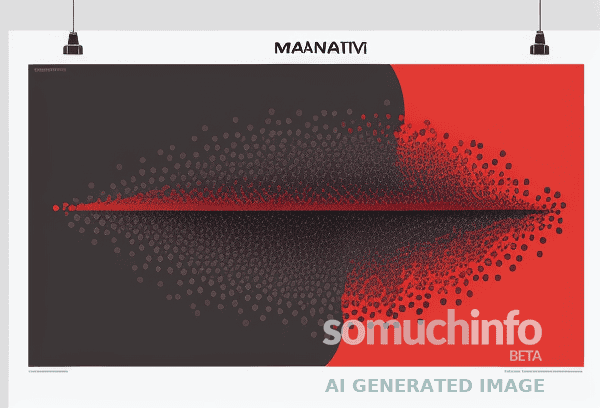
Fisher’s method for determining statistical significance, known as the p-value, became widely adopted. However, this approach had limitations. It didn’t provide a clear way to decide between two hypotheses, and it relied on arbitrary thresholds. This led to criticisms from other statisticians, such as Jerzy Neyman and Egon Pearson.
The Development of Confidence Intervals
Neyman’s innovation was the development of confidence intervals. These intervals provide a range of values within which a population parameter is likely to lie. They address an important question in statistics: how confident can we be about our estimates? However, confidence intervals also have limitations and require careful interpretation.
Jerzy Neyman was a renowned Polish mathematician and statistician.
Born in 1894, he made significant contributions to the field of statistics, particularly in hypothesis testing.
Neyman's work on statistical inference led to the development of the Neyman-Pearson Lemma, a fundamental concept in statistical theory.
He also introduced the concept of confidence intervals and was a pioneer in the application of statistical methods to real-world problems.
The Impact of Fisher’s Legacy
Fisher’s legacy continues to influence statistical analysis today. His approach to determining statistical significance remains widely used, despite its limitations. The relationship between p-values and confidence intervals has also had a lasting impact on the way statistics are presented in medical journals. While there is value in having a measurement that captures uncertainty, it can be misleading to focus solely on specific values.
Moving Forward
As we move forward with statistical analysis, it’s essential to recognize both the benefits and limitations of different approaches. By acknowledging the complexities of statistical inference, we can develop more nuanced methods for interpreting data. This may involve reporting confidence intervals rather than relying solely on p-values. Ultimately, a deeper understanding of statistical principles will help us make more informed decisions in various fields.