A revolutionary new tool, Scale Evaluation, automates the evaluation of state-of-the-art artificial intelligence systems, identifying weaknesses and suggesting additional training data to enhance their performance.
Artificial intelligence (AI) developers are often eager to showcase their latest models as being on the cusp of achieving artificial general intelligence (AGI). However, these models still require significant fine-tuning to reach their full potential.
Scale AI, a prominent player in training and testing advanced AI models, has developed a platform called Scale Evaluation. This tool automates the process of evaluating models across thousands of benchmarks and tasks, identifying weaknesses, and suggesting additional training data to enhance their performance.
The Problem with Human Evaluation
Traditionally, human labor is relied upon to evaluate and fine-tune AI models. Large language models (LLMs) are trained on vast amounts of text scraped from various sources, and turning these models into coherent and well-mannered chatbots requires post-training feedback from humans.
Scale AI supplies workers who specialize in probing models for problems and limitations. The new tool, Scale Evaluation, automates some of this work using Scale’s own machine learning algorithms.
How Scale Evaluation Works
Daniel Berrios, head of product for Scale Evaluation, explains that the platform helps model makers identify areas where their models are not performing well. By analyzing results from various benchmarks and tasks, the tool enables developers to target specific data campaigns for improvement.
One notable example is a case where Scale Evaluation revealed that a model’s reasoning skills deteriorated when fed non-English prompts. The platform highlighted this issue, allowing the company to gather additional training data to address it.
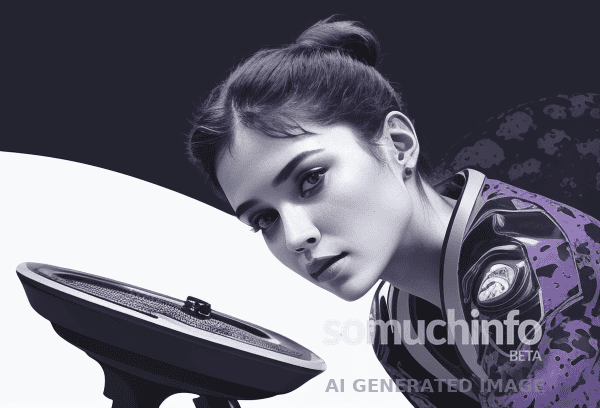
The Future of AI Evaluation
Jonathan Frankle, chief AI scientist at Databricks, sees value in being able to test one foundation model against another. ‘Anyone who moves the ball forward on evaluation is helping us to build better AI,’ he says.
Jonathan Frankle is a computer scientist and researcher at NVIDIA.
He is known for his work on quantization, which involves reducing the precision of neural network weights to reduce memory usage and computational requirements.
Frankle's research has focused on developing techniques for efficient quantization, including quantization-aware training and knowledge distillation.
His work has been published in several top-tier conferences, including NeurIPS and ICLR.
Scale’s new tool offers a more comprehensive picture by combining multiple benchmarks and allowing for custom tests of a model’s abilities. The platform can also generate additional examples to create a more comprehensive test of a model’s skills.
Furthermore, Scale’s tool may inform efforts to standardize testing AI models for misbehavior. A lack of standardization means that some model ‘jailbreaks’ go undisclosed, making it essential to develop methodologies for testing models to ensure they are safe and trustworthy.
Artificial intelligence (AI) safety is a critical concern as machines increasingly perform complex tasks.
Risks include biased decision-making, job displacement, and cybersecurity threats.
To mitigate these risks, researchers focus on developing explainable AI, robustness testing, and value alignment.
Additionally, regulatory frameworks are being established to govern AI development and deployment.
For instance, the European Union's Artificial Intelligence Act aims to ensure transparency, accountability, and human oversight in AI systems.
The Road Ahead
As AI models continue to advance, the need for more efficient evaluation tools becomes increasingly important. Scale Evaluation is a significant step forward in this regard, offering developers a powerful tool for identifying weaknesses and improving their models.
AI evaluation involves assessing an artificial intelligence system's performance, accuracy, and reliability.
This process typically includes testing for bias, fairness, and transparency.
Metrics such as precision, recall, and F1 score are commonly used to measure AI performance.
Additionally, human evaluators assess the system's ability to understand context and make decisions.
The Turing Test is a well-known method for evaluating AI's conversational abilities.