A groundbreaking new approach is transforming the field of pharmaceutical innovation and materials science by harnessing the power of large language models to design molecules with specific properties.
A New Frontier in Medicine and Materials: Can Large Language Models Help?
Understanding the Challenges of Molecule Design
The process of discovering molecules with specific properties is a complex and time-consuming task. It requires vast computational resources and months of human labor to narrow down the enormous space of potential candidates. Large language models (LLMs) like ChatGPT have been proposed as a solution to streamline this process, but enabling an LLM to understand and reason about atoms and bonds has presented a scientific stumbling block.
The Power of Multimodal Models
Researchers from MIT and the MIT-IBM Watson AI Lab have developed a promising approach that combines a large language model with powerful graph-based AI models. This multimodal technique uses a base LLM as a gatekeeper to understand user queries, automatically switching between modules to design molecules, explain rationales, and generate synthesis plans.
Established in 1861, MIT is a private research university located in Cambridge, Massachusetts.
It is known for its programs in science, technology, engineering, and mathematics (STEM).
The university has produced numerous Nobel laureates and has played a significant role in the development of many technologies, including radar, nuclear energy, and artificial intelligence.
How it Works
The LLM predicts text in response to the query, switching between graph modules to generate molecular structures. One module uses a graph diffusion model to create the structure conditioned on input requirements, while another uses a graph neural network to encode the generated structure back into tokens for the LLMs to consume. A final graph module predicts reaction steps.
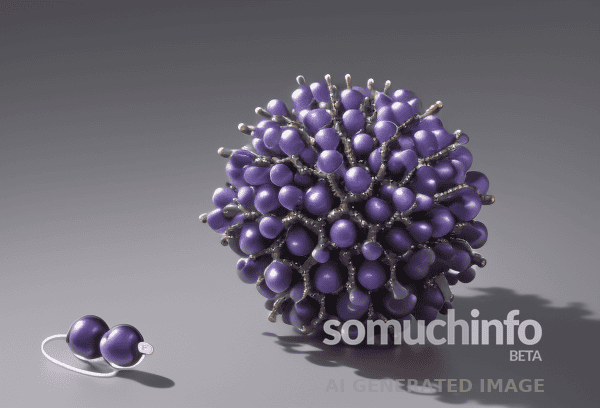
Improved Success Rates
The researchers created a new type of trigger token that tells the LLM when to activate each module. The output of each module is encoded and fed back into the generation process, allowing the LLM to understand what each module did and continue predicting tokens based on those data.
In experiments involving designing molecules that matched user specifications, the multimodal tool outperformed 10 standard LLMs, four fine-tuned LLMs, and a state-of-the-art domain-specific method. It also boosted the retrosynthetic planning success rate from 5 percent to 35 percent by generating molecules with simpler structures and lower-cost building blocks.
Molecules are the smallest units of a substance that retain their chemical properties.
They consist of two or more atoms bonded together through shared electrons.
The number and arrangement of these bonds determine the molecule's structure, which in turn affects its physical and chemical properties.
Molecules can be composed of different elements, such as water (H2O) or carbon dioxide (CO2).
With over 100 million known molecules, they play a crucial role in our daily lives, from the air we breathe to the food we eat.
Future Directions
To generalize Llamole so it can incorporate any molecular property, the researchers plan to improve the graph modules and train the model on more diverse datasets. They hope to use this approach to go beyond molecules, creating multimodal LLMs that can handle other types of graph-based data, such as interconnected sensors in a power grid or transactions in a financial market.
Conclusion
The development of Llamole demonstrates the feasibility of using large language models as an interface to complex data beyond textual description. This research has the potential to revolutionize the field of medicine and materials science, enabling faster and more efficient discovery of new molecules with desired properties.