Smaller language models, with a fraction of the parameters of their larger counterparts, are revolutionizing the field of artificial intelligence by providing more efficient and accessible solutions for various tasks.
The Power of Small: How Reduced Footprint Affects Large Language Models
Large language models have become a staple in the world of artificial intelligence, with their ability to identify patterns and connections making them more powerful and accurate. However, these models come at a cost – they require massive computational resources for training and operation.
The Current State of Large Language Models
Large language models use hundreds of billions of parameters, which are adjusted during the training process to determine connections among data. This allows them to better identify patterns and make more accurate predictions. However, this power comes at a significant cost in terms of energy consumption. A single query to ChatGPT consumes about 10 times as much energy as a single Google search.
The Rise of Small Language Models
In response to the limitations of large language models, researchers are now exploring smaller alternatives. IBM, Google, Microsoft, and OpenAI have all released small language models (SLMs) that use a few billion parameters – a fraction of their larger counterparts. These smaller models excel on specific tasks such as summarizing conversations, answering patient questions, and gathering data in smart devices.
The Benefits of Small Models
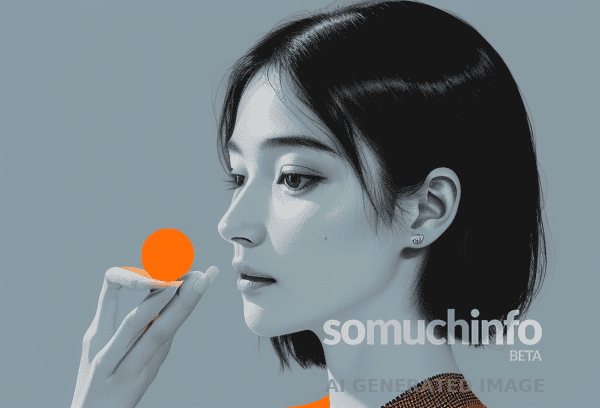
Small models are not designed to be general-purpose tools like their larger cousins but can still achieve impressive results. They require significantly less computational power and can run on a laptop or cell phone, making them more accessible and affordable. The approach used to train these small models is called knowledge distillation, where the larger model effectively passes on its training to the smaller one.
Small language models are a type of artificial intelligence designed to process and generate human-like language. They are smaller in scale compared to large language models, with fewer parameters and less computational power. Despite their limitations, small language models excel in specific tasks such as text classification, sentiment analysis, and machine translation. They require less training data and can be more efficient in resource-constrained environments. Small language models have numerous applications in natural language processing, including chatbots, 'virtual assistants' , and language learning tools .
Optimizing Training for Small Models
Researchers use various techniques to optimize the training process for small models. One method, pruning, involves removing unnecessary or inefficient parts of a neural network, which can help fine-tune a small language model for a particular task or environment. This approach was inspired by the human brain’s ability to gain efficiency by snipping connections between synapses as a person ages.
The Future of Small Language Models
Smaller models offer researchers an inexpensive way to test novel ideas and experiment with new approaches. Because they have fewer parameters than large models, their reasoning might be more transparent. ‘If you want to make a new model, you need to try things,’ said Leshem Choshen, a research scientist at the MIT-IBM Watson AI Lab.
Leshem Choshen is a Talmudic concept referring to the crown of glory that adorns a person's head.
It symbolizes spiritual excellence and is often associated with wisdom, kindness, and humility.
In Jewish mysticism, Leshem Choshen represents the highest level of human consciousness, where an individual's thoughts and actions are guided by divine inspiration.
This concept emphasizes the importance of cultivating inner virtues to achieve a state of spiritual perfection.
Conclusion
The big, expensive models will remain useful for applications like generalized chatbots, image generators, and drug discovery. However, for many users, a small, targeted model will work just as well, while being easier to train and build. These efficient models can save money, time, and compute, making them an attractive alternative for researchers and developers alike.