A new method, self-disciplined autoregressive sampling (SASA), enables large language models to detoxify their own outputs without sacrificing fluency, promoting safer and more ethical language generation.
Large Language Models Can Be Strong Self-Detoxifiers
A new method from the MIT-IBM Watson AI Lab helps large language models steer their own responses toward safer, more ethical, value-aligned outputs. This technique, called self-disciplined autoregressive sampling (SASA), allows LLMs to detoxify their own outputs without sacrificing fluency.
Large Language Models (LLMs) are a type of artificial intelligence designed to process and generate human-like language. They are trained on vast amounts of text data, enabling them to understand context, nuances, and complexities of language. 'They are trained on vast amounts of text data' is a quote that highlights the importance of training data in LLMs. LLMs can perform tasks such as language translation, text summarization, and content generation. They have been widely adopted in applications like chatbots, virtual assistants, and natural language processing systems.
Understanding the Challenge
Large language models naturally contain biases and can generate toxic language. To mitigate this, researchers have explored various methods, including retraining with sanitized datasets and using external reward models. However, these approaches often come with significant computational resources and time requirements. In contrast, SASA leverages the autoregressive nature of LLMs to gradually steer generation away from unsavory or undesired outputs.
Large language models (LLMs) are trained on vast amounts of data, which can introduce biases and stereotypes.
These biases can be reflected in the model's output, perpetuating existing social inequalities.
For instance, studies have shown that LLMs may exhibit gender bias, racial bias, or cultural bias.
This is often due to the data used for training, which may contain discriminatory language or reflect societal prejudices.
To mitigate these issues, researchers are developing techniques to detect and correct biases in LLMs.
The SASA Approach
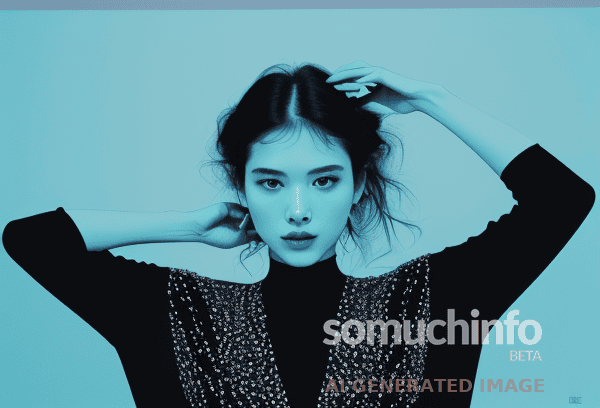
SASA works by building a linear classifier that operates on the learned subspace from the LLM’s embedding. The classifier learns to draw a boundary between toxic and non-toxic subspaces within the sentence embeddings, represented by positive values (non-toxic space) and negative numbers (toxic space). During inference, the algorithm assesses the toxicity value of the partially generated phrase and selects a word option that places the phrase in the non-toxic space.
Evaluating SASA
The researchers evaluated their method against several baseline interventions with three LLMs of increasing size. The results showed that SASA achieved significant toxic language generation reductions, performing on par with state-of-the-art external reward model techniques. However, it was observed that stronger detoxification accompanied a decrease in fluency.
Future Directions
Ko notes that SASA could work well for multiple attributes in the future, such as truthfulness, helpfulness, and loyalty. The technique’s lightweight nature makes it easily applicable to these circumstances, with only marginal overhead in terms of compute and parameters.
Conclusion
SASA represents a significant step forward in developing robust language generation methods that are fair and value-aligned. By leveraging the autoregressive nature of LLMs, SASA offers a fast and efficient way to generate less-toxic language while retaining fluency. As the field continues to evolve, researchers can build upon this work to create more advanced and principled language models.