A groundbreaking study by MIT researchers reveals that the visual pathway in the brain may be processing more than just object recognition, suggesting a complex and multifaceted role for this neural network.
A Visual Pathway in the Brain May Do More Than Recognize Objects
When visual information enters the brain, it travels through two pathways that process different aspects of the input. For decades, scientists have hypothesized that one of these pathways, the ventral visual stream, is responsible for recognizing objects, and that it might have been optimized by evolution to do just that.
How the Ventral Stream Processes Information
The ventral visual stream processes information related to object recognition, including identifying the object, its location, distance from us, and orientation in space. Researchers have used computational models of the anatomy of the ventral stream to investigate how it processes visual information. These models are remarkably good predictors of the neural activities in the ventral stream when optimized for object recognition.
However, a new study by MIT researchers suggests that the ventral stream may not be exclusively optimized for object recognition. Instead, they found that training computational vision models on spatial tasks instead of object recognition resulted in models that were also quite good predictors of the ventral stream’s neural activities.
Training Models to Identify Spatial Features
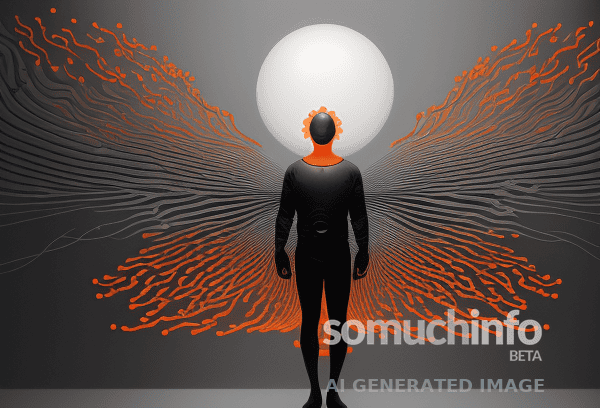
The researchers created a new dataset of synthetic images featuring objects such as tea kettles or calculators superimposed on different backgrounds, in locations and orientations labeled for the model to learn. They trained CNNs to identify one or more spatial features of an object, including rotation, location, and distance.
Surprisingly, these models showed a high level of ‘neuro-alignment’ with the ventral stream, meaning that they were very similar to the neural activities in the brain when trained on just one spatial task. The researchers measured neuro-alignment using a technique developed by DiCarlo’s lab, which involves asking the models to predict the neural activity generated by a particular image.
Neuro-alignment refers to the process of aligning human brain activity with artificial intelligence systems.
This concept has gained significant attention in recent years, particularly in the field of neuroscience and AI research.
Studies have shown that neuro-alignment can be achieved through various methods, including brain-computer interfaces (BCIs) and neural decoding techniques.
Research suggests that successful neuro-alignment can improve human-AI collaboration, enhance cognitive abilities, and potentially treat neurological disorders.
However, the ethics and implications of neuro-alignment remain a topic of debate among experts.
Comparing Models and Understanding Neuro-Alignment
The researchers investigated why training for object recognition and training for spatial features led to similar degrees of neuro-alignment. They used an analysis known as centered kernel alignment (CKA), which measures the degree of similarity between representations in different CNNs.
The results showed that in the early to middle layers, the representations learned by these models were nearly indistinguishable. The researchers hypothesize that even when models are trained to analyze just one feature, they also take into account ‘non-target’ features – those that they are not trained on.
This suggests that the models are using all available information to learn a better representation, which may result in different models coming up with similar representations.