Revolutionizing complex planning problems, MIT researchers introduce learning-guided rolling horizon optimization (L-RHO), a method that harnesses machine learning to predict which operations should be recomputed, significantly reducing solve times while maintaining solution quality.
A Faster Way to Solve Complex Planning Problems
Complex planning problems have long been a challenge for industries such as transportation, logistics, and manufacturing. Traditional approaches often rely on manual optimization techniques that can be time-consuming and prone to errors.
The Problem of Redundant Computations
One major issue with traditional solvers is the need for redundant computations. When solving complex combinatorial problems, many decisions are repeated unnecessarily, which can lead to slow solve times and suboptimal solutions.
A New Approach: Learning-Guided Rolling Horizon Optimization
To address this problem, MIT researchers have developed a new method called learning-guided rolling horizon optimization (L-RHO). This approach uses machine learning to predict which operations should be recomputed when the planning horizon rolls forward. By freezing certain variables and only reoptimizing those that are necessary, L-RHO can significantly reduce solve times while maintaining solution quality.
Learning-Guided Rolling Horizon Optimization (LG-RHO) is a dynamic scheduling approach that combines machine learning with traditional optimization techniques. It involves breaking down complex problems into smaller, manageable chunks, and using historical data to inform future decisions. LG-RHO uses rolling horizon planning, where the optimization problem is solved for a limited time period, and then updated based on new information. This approach enables flexible response to changing conditions and improves overall system performance.
How it Works
The L-RHO method involves two main components: a classical algorithmic solver and a machine-learning model. The solver is used to break down the problem into smaller subproblems, which are then solved using traditional optimization techniques. Meanwhile, the machine-learning model learns from these subproblems to predict which operations should be recomputed when the planning horizon advances.
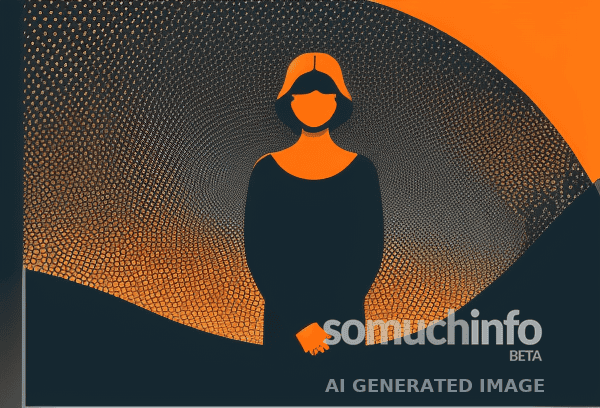
Machine learning is a subset of artificial intelligence that enables systems to learn from data without being explicitly programmed.
This field has its roots in the 1950s, with the development of the first neural networks.
In the 1980s, machine learning gained popularity with the introduction of decision trees and support vector machines.
The advent of deep learning in the 2000s revolutionized the field, enabling systems to learn complex patterns in large datasets.
Today, machine learning is applied in various domains, including image recognition, natural language processing, and predictive analytics.
Advantages of L-RHO
L-RHO has several advantages over traditional approaches. It can reduce solve times by up to 50% and improve solution quality by up to 21%. Additionally, the method is adaptable and scalable, making it suitable for a wide range of complex optimization problems.
Real-World Applications
The L-RHO method has the potential to be applied in various industries, including transportation, logistics, manufacturing, and more. By reducing solve times and improving solution quality, L-RHO can help companies make faster and more informed decisions about resource allocation, scheduling, and planning.
Transportation has undergone significant transformations throughout history, from ancient civilizations using camels and horses to modern times with electric vehicles and hyperloops.
In the late 19th century, the invention of the automobile revolutionized personal transportation.
The 20th century saw the rise of air travel, with commercial flights becoming accessible to the masses.
Today, emerging technologies like self-driving cars and high-speed rail continue to shape the future of transportation.
Future Research Directions
While L-RHO has shown promising results, there is still room for improvement. The researchers hope to better understand the logic behind their model’s decision to freeze certain variables and not others. They also plan to integrate their approach into other types of complex optimization problems, such as inventory management and vehicle routing.
Conclusion
The development of L-RHO represents a significant breakthrough in the field of machine learning and optimization. By harnessing the power of artificial intelligence to reduce redundant computations, this method has the potential to transform industries and revolutionize the way we approach complex planning problems.