A Harvard research initiative is using physics and neuroscience to uncover the fundamental principles that drive artificial intelligence’s learning process, aiming to improve A.I. systems and minimize bias.
A group of Harvard researchers is using physics and neuroscience to study artificial intelligence’s internal logic, aiming to uncover the fundamental principles that drive its learning and reasoning.
Artificial intelligence (AI) has a rich history dating back to 1951 when computer scientist Alan Turing proposed the Turing Test, a measure of a machine's ability to exhibit intelligent behavior equivalent to, or indistinguishable from, that of a human.
Since then, AI research has accelerated with significant advancements in machine learning, natural language processing, and deep learning.
Today, AI is transforming industries such as healthcare, finance, and transportation with applications like predictive maintenance, personalized medicine, and autonomous vehicles.
The team, led by Hidenori Tanaka, has launched the Physics of Artificial Intelligence (PAI) Group at Harvard University’s Center for Brain Science. By applying principles from physics to understand how A.I. learns, they hope to identify the laws that govern its internal logic.
Understanding the Limitations of Benchmarking
Currently, the capabilities of an A.I. are measured through benchmarking, which typically involves testing models against a set of standardized tasks or problems. However, Tanaka believes this method is limited and fails to capture the cognitive depth of A.I. models. ‘We need to go beyond benchmarking,’ he said. ‘It’s an insult to judge A.I. models based on mere computational power and how well they solve a couple of tough problems.‘
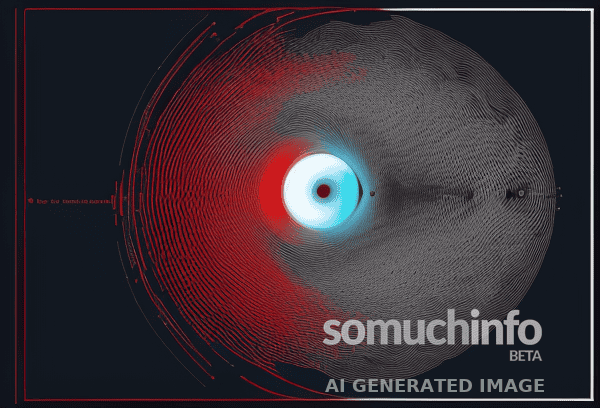
Building Controlled Digital Experiment Environments
To achieve this, PAI is building ‘model experimental systems’ – controlled digital experiment environments that allow developers to observe how an A.I. model’s learning and reasoning curve evolves over time. The team is crafting numerous multimodal datasets consisting of images and text across various topics, including physics, chemistry, biology, math, and language.
These datasets are intentionally crafted with distinct, predefined functions, unlike internet-scraped data. By partnering with A.I. developers worldwide, PAI aims to improve these datasets through insights gleaned from real-world experiments. ‘The goal is to give A.I. systems a structured playground,’ Tanaka explained. ‘Just like medications act on specific neurons to treat a physical condition in humans, we’re looking at how information triggers responses within A.I. models at the neural or node level.‘
A Collaborative Effort
PAI’s core team includes multiple Harvard researchers and collaborates with experts from other institutions, including neuroscientist Venkatesh Murthy, Princeton professor Gautam Reddy, and Stanford’s Surya Ganguli. The group has delivered over 150 papers in A.I. research and has one of its previous research projects on neural network pruning algorithms cited over 750 times.
By studying the brain’s computational principles and aligning them with physical laws, PAI hopes to unlock the secrets of artificial intelligence’s learning process. This research has the potential to improve A.I. systems, minimize bias, and reduce hallucinations in upcoming models.