A new computational model, React-OT, has been developed to predict the point of no return in chemical reactions, enabling chemists to design more efficient reactions and yield useful compounds.
New Model Predicts Chemical Reaction’s Point of No Return
Chemists could use this quick computational method to design more efficient reactions that yield useful compounds, from fuels to pharmaceuticals.
A chemical reaction is a process where one or more substances, called reactants, are converted into new substances, called products.
This transformation occurs through the interaction of atoms and molecules, resulting in changes to their chemical composition.
Chemical reactions can be spontaneous or triggered by external factors such as heat, light, or catalysts.
They play a vital role in various natural processes like digestion and respiration, as well as industrial applications like manufacturing and energy production.
The Challenge of Transition States
When chemists design new chemical reactions, one useful piece of information involves the reaction’s transition state—the point of no return from which a reaction must proceed. This information allows chemists to try to produce the right conditions that will allow the desired reaction to occur. However, current methods for predicting the transition state and the path that a chemical reaction will take are complicated and require a huge amount of computational power.
A Breakthrough in Computational Chemistry
MIT researchers have now developed a machine-learning model that can make these predictions in less than a second, with high accuracy. Their model could make it easier for chemists to design chemical reactions that could generate a variety of useful compounds, such as pharmaceuticals or fuels.
Massachusetts Institute of Technology (MIT) is home to a community of renowned researchers who have made groundbreaking contributions to various fields, including science, technology, engineering, and mathematics.
With a strong focus on interdisciplinary research, MIT researchers have developed innovative solutions to complex problems, improving lives globally.
Notable examples include the discovery of gravitational waves by the LIGO Scientific Collaboration and the development of the first practical battery by John Goodenough.
Their work has earned them numerous awards, including Nobel Prizes and National Medals.
How the Model Works
The new model, React-OT, uses a different strategy than previous models. It begins from an estimate of the transition state generated by linear interpolation—a technique that estimates each atom’s position by moving it halfway between its position in the reactants and products, in three-dimensional space. This approach allows the model to start with a better initial guess than just a completely random guess.
Improved Accuracy and Speed
Because of this, React-OT takes fewer steps and less time to generate predictions. In the new study, the researchers showed that their model could make predictions with only about five steps, taking about 0.4 seconds. These predictions don’t need to be fed through a confidence model, and they are about 25 percent more accurate than the predictions generated by the previous model.
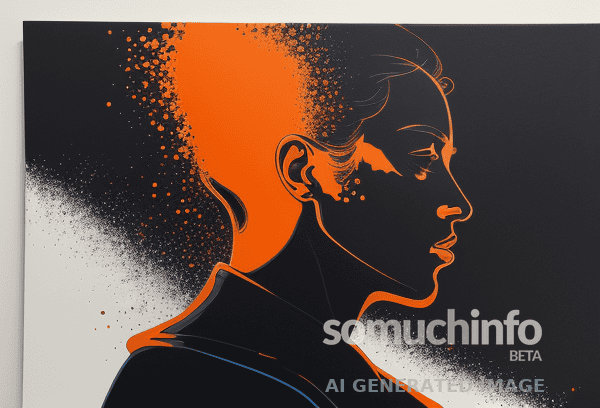
Potential Applications
The researchers’ new model has the potential to accelerate our search and optimization processes in computational chemistry. By quickly predicting transition state structures, chemists can design reactions that are more efficient and yield useful compounds. The MIT team hopes that other scientists will make use of their approach in designing their own reactions.
Generalizing Across Different System Sizes
The React-OT model performs well on a wide array of chemistry, including polymerization reactions where the reaction is occurring in just one part. This means that it can tackle a broad range of chemical systems, from small organic or inorganic molecules to larger reactants with side chains.
Future Directions
The researchers are now working on training the model so that it can predict transition states for reactions between molecules that include additional elements, such as sulfur, phosphorus, chlorine, silicon, and lithium. This could accelerate our search and optimization processes even further.
Accelerating Chemical Understanding
To quickly predict transition state structures is key to all chemical understanding, says Markus Reiher, a professor of theoretical chemistry at ETH Zurich. The new approach presented in the paper could very much accelerate our search and optimization processes, bringing us faster to our final result. As a consequence, also less energy will be consumed in these high-performance computing campaigns. Any progress that accelerates this optimization benefits all sorts of computational chemical research.
A Tool for Designing Chemical Reactions
The MIT team has created an app for designing chemical reactions using the React-OT model. With this tool, users can input a reactant and product, and the model will generate the transition state from which they can estimate the energy barrier of their intended reaction and see how likely it is to occur.
Funding and Future Directions
The research was funded by the U.S. Army Research Office, the U.S. Department of Defense Basic Research Office, the U.S. Air Force Office of Scientific Research, the National Science Foundation, and the U.S. Office of Naval Research. The MIT team hopes that their approach will have a significant impact on computational chemistry and inspire other scientists to develop new models and methods for designing chemical reactions.
The MIT team is a group of students, researchers, and faculty members from the Massachusetts Institute of Technology.
Known for their 'innovative spirit' and academic excellence, the team has made significant contributions to various fields, including science, technology, engineering, and mathematics (STEM).
With a strong focus on research and development, the MIT team has produced numerous patents, publications, and awards in areas such as artificial intelligence, biotechnology, and renewable energy.