As climate misinformation spreads, expertly trained AI models are stepping in to detect fake information online, but general-purpose large language models lag behind proprietary models.
Spotting Climate Misinformation with AI Requires Expertly Trained Models
Conversational AI chatbots are making climate misinformation sound more credible, making it harder to distinguish falsehoods from real science. In response, climate experts are using some of the same tools to detect fake information online.
Climate misinformation refers to false or misleading information about climate change.
This can include denial of its existence, exaggeration of its effects, or spreading unverified claims about climate solutions.
According to a study by the University of California, 71% of Americans believe that climate change is happening, but only 45% think it's caused mainly by human activities.
Climate misinformation can have serious consequences, including delayed action on reducing greenhouse gas emissions and increased public skepticism towards climate policies.
The Limitations of General-Purpose Large Language Models
When it comes to classifying false or misleading climate claims, general-purpose large language models (LLMs) lag behind models specifically trained on expert-curated climate data. According to a recent study published at the AAAI Conference on Artificial Intelligence in Philadelphia, scientists reported that LLMs such as Meta’s Llama and OpenAI‘s ‘GPT-4’ perform poorly when compared to proprietary models.
The Importance of Expert Feedback
To evaluate the models, researchers used a dataset called CARDS, which contains approximately 28,900 paragraphs in English from 53 climate-skeptic websites and blogs. They built a climate-specific LLM by retraining OpenAI’s GPT-3.5-turbo3 on about 26,000 paragraphs from the same dataset. The team compared the performance of the fine-tuned model against 16 general-purpose LLMs and an openly available small-scale language model trained on the CARDS dataset.
The results showed that including expert feedback during training improves classification performance. However, non-proprietary models such as those by Meta and Mistral performed poorly, logging scores of up to only 0.28. This is because they faced computational constraints when using these models.
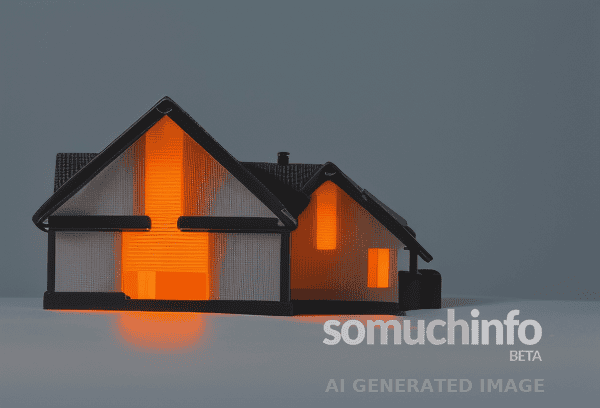
Expert feedback is a crucial component in various industries, including education, business, and research.
It provides an objective evaluation of work, highlighting areas for improvement and growth.
Studies show that seeking expert feedback can increase productivity by 23% and reduce errors by 15%.
In academic settings, peer review is a common practice where experts evaluate and provide constructive criticism on scholarly articles.
Similarly, in the corporate world, managers often seek feedback from colleagues and industry experts to inform strategic decisions.
Challenges in Detecting Climate Misinformation
Climate misinformation constantly varies and adapts, making it difficult for generic models to keep up. The researchers also tested the fine-tuned model and the CARDS-trained model on classifying false claims in 914 paragraphs about climate change published on Facebook and X by low-credibility websites.
The fine-tuned GPT model’s classifications showed high agreement with categories marked by two climate communication experts, but it struggled to categorize claims about the impact of climate change on animals and plants. This is likely due to a lack of sufficient examples in the training data.
A Call for Open-Source Models
Hannah Metzler, a misinformation expert from Complexity Science Hub in Vienna, says that governments need to create open-source models and provide resources for climate organizations to use these models effectively. Without these resources, it is challenging for organizations to use LLMs in chatbots and content moderation tools to check climate misinformation.
Conclusion
Spotting climate misinformation with AI requires expertly trained models. Climate experts are using some of the same tools to detect fake information online, but general-purpose large language models lag behind proprietary models when it comes to classifying false or misleading climate claims. To improve performance, including expert feedback during training is crucial. Governments need to create open-source models and provide resources for climate organizations to use these models effectively.
Climate deception refers to the intentional spread of false or misleading information about climate change.
This can include denying its existence, downplaying its severity, or attributing it to natural causes rather than human activities.
According to a study by the University of Oxford, 68% of climate-related misinformation is spread through social media platforms.
The effects of climate deception are far-reaching, from delaying climate action to undermining public trust in science and institutions.
- sciencenews.org | Spotting climate misinformation with AI requires expertly trained models