Breakthrough computational chemistry method accelerates molecular insights with neural networks, transforming materials science and opening doors to novel materials with desirable properties.
Accelerating Materials Design with Neural Networks
A New Frontier in Computational Chemistry
Computational chemistry has come a long way since the old days of alchemy. With the advent of machine learning tools, researchers have been able to determine the structure and physical properties of various molecules and substances with greater accuracy. However, there is still room for improvement. A new approach developed by MIT researchers uses neural networks to accelerate the prediction of molecules and materials.
The Challenges of Quantum Mechanics
Quantum mechanics provides a quantum mechanical approach to determining the total energy of a molecule or crystal by looking at the electron density distribution. While this method has been successful, it has some drawbacks: accuracy is not uniformly great, and it only tells you one thing – the lowest total energy of the molecular system. The problem is that carrying out these calculations on a computer is very slow, and the scaling is bad.
A Novel Approach
To overcome these challenges, MIT researchers have developed a multi-task machine learning approach to predict the electronic properties of molecules. They use a novel architecture called the Multi-task Electronic Hamiltonian network (MEHnet), which sheds light on a number of electronic properties, such as the dipole and quadrupole moments, electronic polarizability, and the optical excitation gap.
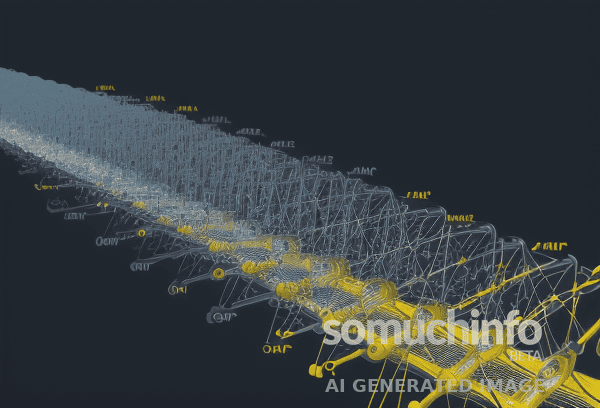
The Power of Machine Learning
The MEHnet model is trained using coupled-cluster theory (CCSD(T)) calculations, which are much more accurate than what you get from density functional theory (DFT) calculations. However, CCSD(T) calculations are slow and scale badly with the number of electrons in the system. The machine learning model can perform these same calculations much faster by taking advantage of approximation techniques.
Applications and Future Directions
The MIT-based group has applied their model to small, nonmetallic elements and has since moved on to examining heavier elements. They believe that this approach holds the potential for high-throughput molecular screening, which is essential for identifying novel molecules and materials with desirable properties. The future direction of this research is wide open, with the ambition to cover the whole periodic table with CCSD(T)-level accuracy at lower computational cost than DFT.
Implications for Materials Science
This new approach has significant implications for materials science. It can be used to characterize molecules that haven’t been seen before and predict the properties of hypothetical materials. The examination of heavier transition metal elements could lead to the advent of new materials for batteries, an area of acute need.
Conclusion
The development of a multi-task machine learning approach to accelerate the prediction of molecules and materials is a significant breakthrough in computational chemistry. With its potential for high-throughput molecular screening and its ability to cover the whole periodic table with CCSD(T)-level accuracy at lower computational cost than DFT, this research has the power to transform the field of materials science.