OpenAI’s latest AI research tool, ‘Deep Research,’ has sparked both excitement and concern with its ability to generate in-depth analysis and distinguish fact from speculation. However, critics argue that the technology’s impressive capabilities come with significant caveats, including the potential for factual inaccuracies and a lack of rigor in its findings.
OpenAI has unveiled a new research tool called ‘deep research‘ that can pull information from the web and summarize it into detailed reports, supposedly at the level of a research analyst. However, this impressive technology comes with significant caveats.
The Capabilities of Deep Research
“Deep research is a type of AI agent designed to perform tasks on behalf of its users.” It leverages an upcoming version of OpenAI’s o3 model and can process text, images, and PDFs on the internet in tens of minutes, accomplishing what would take a human many hours. This tool also features an activity sidebar that shows a step-by-step summary of its process in real time.
Deep research involves thoroughly examining a topic through various sources and methods to gain a comprehensive understanding.
This approach helps identify patterns, relationships, and gaps in knowledge.
According to a study by the National Science Foundation, 70% of researchers believe that deep research improves the quality of their work.
Deep research also enables scientists to validate or refute existing theories and develop new ones.
It is an essential component of academic and professional endeavors.
Availability and Features
Deep research is available only to those who are subscribed to the $200-per-month ChatGPT Pro plan. Its final reports are strictly text-based, but OpenAI plans to add images and data visualizations in the coming weeks. While it can provide hyper-personalized recommendations on big purchases like cars and appliances, its primary focus is on ‘intensive knowledge work’ in fields like finance and science.
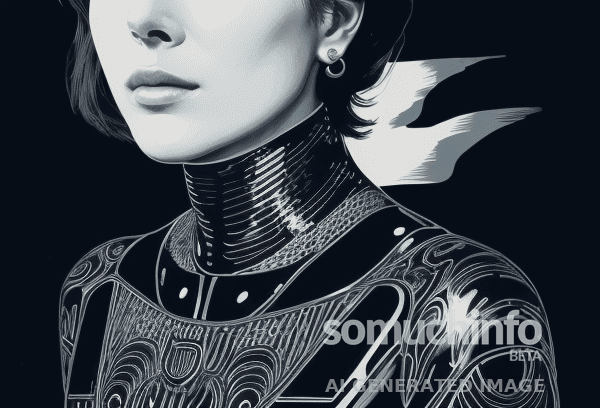
The Drawbacks of Deep Research
Despite its impressive capabilities, deep research has significant drawbacks. Like all large language models, it can sometimes “hallucinate – or make up” facts, although OpenAI claims this occurs at a lower rate than with existing models. The AI agent also struggles to separate authoritative information from rumors and often fails to convey uncertainty, passing fiction off as fact.
Hallucinations in AI refer to instances where a machine learning model generates outputs that are not supported by the input data.
This phenomenon occurs when models overfit or misinterpret training data, leading to unrealistic or fabricated information.
Studies have shown that hallucinations can be caused by biases in training datasets, inadequate regularization techniques, and flawed architecture design.
According to research, around 30% of AI-generated text exhibits hallucinatory behavior.
To mitigate this issue, developers employ techniques such as data augmentation, adversarial training, and attention-based mechanisms.
Rigor of Research and Trustworthiness
The lack of rigor in deep research’s findings raises concerns about its trustworthiness. Users may need to spend significant time double-checking the accuracy of its synthesized reports and the sources it cites. This is particularly problematic given that deep research is explicitly geared towards serious knowledge gathering, even in scientific fields.
OpenAI’s Rushed Development
The release of deep research follows OpenAI’s recent AI agent, Operator, which was showcased last month for its ability to shop for groceries and make reservations online. The company’s rapid development pace has sparked questions about the thoroughness of its testing and validation processes.