A recent study has revealed that large language models process diverse data types through a ‘semantic hub’ mechanism, mirroring the human brain’s ability to integrate information from various modalities.
A recent study has shed light on how large language models (LLMs) process diverse data types, revealing similarities between their internal workings and those of the human brain. Researchers from MIT have found that LLMs use a ‘semantic hub’ mechanism to integrate information from various modalities, such as text, images, audio, and even computer code.
Large language models are artificial intelligence systems that use neural networks to process and generate human-like language.
These models have gained significant attention in recent years due to their ability to understand and respond to complex queries.
They are trained on vast amounts of text data, allowing them to learn patterns and relationships within language.
This enables them to perform tasks such as language translation, text summarization, and even generating coherent paragraphs.
According to a study by OpenAI, large language models have achieved state-of-the-art results in various natural language processing tasks.
The study builds upon previous work that suggested English-centric LLMs rely on English to perform reasoning processes on other languages. The researchers expanded this idea by probing the inner workings of LLMs using a range of experiments. They found that LLMs process data in their specific language or modality, but then convert tokens into modality-agnostic representations as they reason about them throughout their internal layers.
The researchers believe that this semantic hub strategy is similar to how the human brain integrates diverse information from various modalities. In the human brain, a ‘semantic hub’ in the anterior temporal lobe integrates semantic information from visual data and tactile inputs. This hub is connected to modality-specific ‘spokes’ that route information to the hub.
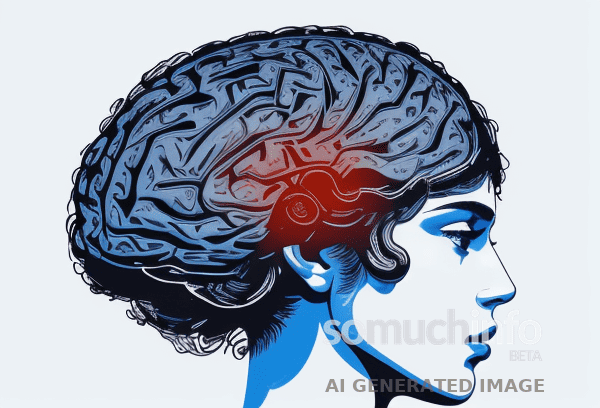
The human brain is a complex and intricate organ that weighs approximately 1.4 kilograms (3 pounds) in adults.
It consists of billions of neurons, which are connected by trillions of synapses.
The brain's cerebral cortex is responsible for processing sensory information, controlling movement, and facilitating thought, emotion, and memory.
The brain also contains the cerebellum, which coordinates muscle movements, and the brainstem, which regulates basic functions such as breathing and heart rate.
These findings have significant implications for future research on LLMs. By understanding how they process diverse data, scientists can develop more efficient models that share knowledge across languages and modalities. However, researchers also acknowledge that there may be concepts or knowledge that are not translatable across languages or data types, requiring language-specific processing mechanisms.
The study’s insights could lead to improved multilingual models, which often lose accuracy in their dominant language when learning other languages. By better understanding an LLM’s semantic hub, researchers can prevent language interference and create more effective models for a range of applications.
Multilingual models are artificial intelligence systems designed to understand and process multiple languages simultaneously.
These models can learn patterns, relationships, and nuances between languages, enabling more accurate translations and improved language understanding.
According to a study, multilingual models have shown an average improvement of 20% in translation accuracy compared to monolingual models.
By leveraging multilingual models, businesses can expand their global reach, improve customer engagement, and increase revenue.
The researchers propose exploring how to maximize the sharing of information across diverse data types while allowing for language-specific processing mechanisms. This could involve developing new model architectures that leverage the semantic hub strategy.